Research in life sciences is entering a period of disruption in how scientific data are collected and analyzed. BioTeam’s CEO, Dr. Ari Berman, discusses why scientific organizations that build integrated data ecosystems will pioneer new discoveries the fastest. The article was originally published in Genetic Engineering & Biotechnology News‘ 40th anniversary issue of their online magazine.
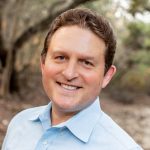
To derive value from fast-growing data collections, research organizations are using high-performance platforms, exploring standards, and prioritizing FAIRness
Research in the life sciences, biotechnology, and biomedicine is entering a period of disruption in how scientific data are collected and analyzed. This disruption is having widespread impacts since data have always been central to research. Consider how the typical research project progresses: a problem is defined; a study is conceived (and hopefully funded); experiments are run; data are collected, analyzed, interpreted, and used to support conclusions; and conclusions are communicated to select audiences or the broader scientific public.
In research, data connect the experiment and the generation of knowledge. Increasing the speed of that process is more important than ever. Quality of life can be improved, and lives saved as a result—as the COVID-19 pandemic has illustrated so vividly.
Disruptive scientific innovation requires organizations to
transform petabytes of data into a strategic asset
Wrangling data has become more challenging because of the enormous growth in the production of scientific data. Disruptive scientific innovation requires organizations to transform these petabytes of data into a strategic asset by making them findable, accessible, interoperable, and reusable (FAIR). Organizations that build effective scientific data ecosystems to harness the knowledge inherent in their data will pioneer new discoveries the fastest.
For the past 20 years, biomedical research has been utilizing laboratory technologies that generate unprecedented amounts of data in a short time. This increased rate of data generation has been primarily driven by the pace of innovation in laboratory technology. These data are produced by instruments such as high-throughput genomic sequencers, next-generation fluorescent microscopes (like lattice light-sheet microscopes), cryo-electron microscopes, flow cytometers, and a host of other imaging, resonance, and data collection technologies.
By applying these technologies, researchers hope to dig deeper into the problems that plague humanity, find new disease treatments, and realize exciting concepts such as precision and personalized medicine. These technologies are inspiring researchers to launch masterful studies and collect data that may, upon analysis, help us chip away at the mystery of life.
This explosion of data collection led to the information age and the coining of ill-defined buzz terms like “big data.” An unintended consequence of this change was that information technology (IT) personnel in life sciences organizations were caught off guard. They suddenly had to support massive amounts of data (a petabyte in 2012, hundreds of petabytes today) without the necessary budgets, skill sets, or support systems.
Prior to this data tsunami, IT groups mostly supported document storage, databases, email, web, security, and printers. Now that the data deluge is upon us, aspects of the hard-earned expertise of the high-performance computing (HPC, also known as supercomputing) community are slowly being adopted to help them adapt.
Life sciences organizations began to invest in scientific computing by building modest to large HPC systems, installing large storage systems, and working to better connect laboratories to data centers so that data could flow more easily. These adaptations took many years and occurred at varying rates per organization. Ultimately, though, the data center and advanced computing technologies became as integral to life sciences research as a microscope or a next-generation sequencer.
Modern research projects cannot be done without an advanced technology infrastructure. HPC, storage systems, high-speed networks, and public cloud environments have become essential lab tools, not merely devices for IT to operate and maintain.
Drowning in data
Today, the scientific community confronts a data landscape that is not just more expansive, but also more varied. There are now vast repositories of scientific data and organizations creating every conceivable type of data architecture (i.e., data lakes, oceans, fogs, and islands), culminating in the growth of data commons as a fundamental scientific data architecture. With innovations in data science and bioinformatics, it may be possible to start discussing how the current pace of data accumulation can be maintained. One possibility is the development and adoption of common data standards for biomedical data.
However, most researchers in our field are still creating their own data formats and metadata assignments while putting their data wherever they think is best for their research. Without effective standards, high-value data are being spread across every data storage medium imaginable, including portable disks that end up being shoved into drawers. IT is unable to keep up with hardware acquisition, and the data are piling up stochastically.
The backlog of data has led most biomedical organizations to turn to public cloud providers (such as Amazon Web Services, Google Cloud, and Microsoft Azure) to alleviate their on-premises logjams. Public clouds have also proven highly beneficial for collaborative data analytics by placing data and computational resources in close proximity outside of the security restrictions local enterprise networks place at their borders.
However, the sprawl of data, the backlog of data analysis, and the difficulty of combining multiple datasets for more detailed studies have led to the realization that collecting data alone is not useful. To give value to the data, it needs to be analyzed, interpreted, and converted into knowledge for the community to consume. This realization has led the life sciences community to the end of the information age and into the analytics age.
Much of the world has turned to artificial intelligence (AI), specifically machine learning (ML) and deep neural networks, to solve the problem of interpreting large and potentially unstructured datasets. The hope is that by creating inference models that represent the data, it will become possible to perform analyses more quickly and to assign meaning more easily. This methodology, which has been hyped by hardware and software vendors for the last few years through marketing campaigns, had the positive consequence (perhaps unintended) of driving a desperate field to explore its viability and has resulted in several important innovations in data science.
Unfortunately, there are still several issues facing the life sciences community. First, without unified data standards and common approaches to data governance, data will never become FAIR—an end goal for the community to efficiently utilize public data with any other research project. Additionally, well-curated data tagged with common and actionable metadata that clearly define what the data represent are needed to create the necessary datasets to train the ML models. If the data aren’t clear, ML models will not be useful.
Additionally, despite the claims by the industry, deep learning is not the magic bullet algorithm that will save everyone from their data sprawl. It is helpful only in certain situations and only when data is well curated.
Once the life sciences community gets a better hold on its data, starts progressing toward unified data standards, and accomplishes FAIRness to a meaningful degree, we’ll begin to understand the actual value of the collected data. We’ll make more informed choices about which data to keep and what qualifies as intermediate or lower value items in storage schemes. If we can slow the exponential growth of data, start managing the backlog, and work toward common data platforms (data commons are a good start), we’ll establish true scientific data ecosystems across the industry.
Digital transformation
The process of working toward a well-established and functional scientific data ecosystem is called “digital transformation,” another buzz term. For digital transformation to be successful, the scientific data ecosystem must be designed with a holistic approach aligned with the organization’s scientific mission with advanced technology at its core.
Our company, BioTeam, has been working with large organizations for the last several years building up digital transformation strategies and platforms. Starting with the National Institutes of Health, we’ve been working with the Office of Data Science Strategy (ODSS) facilitating collaboration with the Institutes and Centers at NIH to form a large data ecosystem out of the many data repositories that NIH funds and maintains.
We’ve also been working with large pharmaceutical companies to make better use of their data by improving their IT infrastructure to support science, creating collaborative scientific computing environments in the cloud, and building data commons customized to the needs of the organization. The most public of these efforts has been the development of an internal data commons for Bristol Meyers Squibb (BMS) Research and Early Development using the open source Gen3 framework (maintained by the University of Chicago) as the foundation for the system.
Most biotechnology and pharmaceutical companies that we work with are going through various stages of this transformation. Academic institutions and federal science agencies are all either planning or already working to implement some or part of a digital transformation strategy.
The establishment of productive scientific data ecosystems is within our reach, but it will require unprecedented collaboration. To help foster community-wide agreement, global governing bodies may need to offer incentives and put enforcement mechanisms in place. As terrible as the COVID-19 pandemic has been, it taught us a valuable lesson about the value of collaboration. It proved the need for this kind of coordination as researchers and scientists around the world attempted to rapidly work together to mount a response against this novel and devastating disease.
The aforementioned barriers proved to be profoundly challenging to overcome during the pandemic response, even with public clouds, supercomputing centers, and other organizations donating and prioritizing use of their resources to anyone working on the problem. The lack of an established scientific data ecosystem has drastically curtailed our progress. Nonetheless, this is a truly exciting time in our field as we transition into the analytics age. Let’s work together. Let’s change the culture in the life sciences, biotechnology, and biomedical research. And let’s build lasting scientific data ecosystems that will drive our understanding of life on Earth to the next level.
Want to read more great content from BioTeam? Subscribe to our newsletter for quarterly updates.